The RSA Conference 2024 is set to kick off on May 6. Known as the “Oscars of Cybersecurity”, RSAC Innovation Sandbox has become a benchmark for innovation in the cybersecurity industry.
Let’s focus on the new hotspots in cybersecurity and understand the new trends in security development.
Today, let’s get to know the company Bedrock Security.
Introduction of Bedrock Security
Bedrock Security is a company focused on data security, with a particular emphasis on data security in the cloud and GenAI era. Their mission is to enable organizations to confidently manage data growth without compromising security.
Founded in 2022, Bedrock Security was established by data security experts Pranava Adduri and Ganesha Shanmuganathan.
Pranava Adduri previously served as a founding engineer at unicorn startups Box and Rubrik, and he expanded a new product line to over $200 million at Amazon Web Services (AWS). He is passionate about helping organizations protect user data. During his tenure at Rubrik and AWS, Adduri collaborated with Fortune 500 clients to establish reliable, scalable data protection and security services. His solutions helped Fortune 500 companies mitigate catastrophic ransomware attacks.
Ganesha Shanmuganathan has an engineering background from Cohesity and VMware, with over 50 patents. He and Adduri jointly developed the Bedrock platform.
In March 2024, Bedrock Security announced securing a $10 million seed fund led by Greylock[1] . Greylock has previously invested in cybersecurity companies such as Palo Alto Networks, Okta, Obsidian Security, and Opal.
Background Introduction
To adapt to competition and market demands, businesses are rapidly adopting cloud computing and GenAI services, leading to explosive growth in data that exceeds current data security capabilities. To address the security issues brought about by the growing data, businesses need to continually evolve and develop their data security capabilities. They must ensure the accurate identification of these massive amounts of data and protect the security of this data without impeding business operations.
The rapid growth of data and increasingly complex network environments have brought about three data security challenges:
- Rapidly growing and moving data volumes: It is very difficult to discover sensitive data and classify it, especially when data grows, moves, and replicates in a distributed environment.
- Structured and unstructured data types: Structured data is easier to analyze and classify than unstructured data, but most companies have both types of data. Traditional data security solutions are very rigid in identifying data types, making it difficult to provide appropriate protection.
- Dealing with massive amounts of data: Analyzing massive amounts of data is often slow, inaccurate, and expensive. To maintain accuracy, frequent rescanning is required, but the rapid growth of data volumes and slow analysis times hinder the processing of massive data. Fixed sampling only looks at a small part of all data, which is much faster but much less accurate, resulting in sensitive data not being protected.
Traditional Data Security Can’t Meet Today’s Challenges
Despite data growing exponentially, corresponding security measures are growing linearly – security teams cannot grow in size according to data volume. According to IDC forecasts, global data volume will reach 175 zettabytes (ZB) by 2025. A survey shows that an organization deals with 63 percent growth in data volume per month, on average, with 12 percent of respondents reporting 100 percent growth[2] . At the same time, organizations are facing an increasing number of network threats against this data.
Existing traditional security tools are not specifically designed for analyzing and protecting organization data use. The frequency of attacks is increasing, and the attack surface is expanding with the increase in data. There are more and more regulations related to data security[3] , requiring companies affected by network incidents to respond in a shorter time. Due to various reasons, including a lack of cybersecurity talent[4], the response may not be as rapid. Delays in identifying and classifying sensitive data can lead to longer recovery, repair, response, or resolve.
Security teams must quickly identify and classify data, and reinforce it as much as possible to ensure that data access complies with regulations, without excessive data access permissions or data exposure. At the same time, security teams need to continuously assess data security events that violate policies. Delays in data access create friction between security and other business lines, with different teams wanting to access data first, leading to competition for resources, and more investment in data security. The constantly growing data demand poses new challenges to security teams, which may reduce their ability to respond to other network threats and security issues.
Traditional data security solutions, including Data Security Posture Management (DSPM), cannot meet modern business needs. Traditional data security solutions often rely on (inaccurate) rule-based data classification, which cannot guarantee accuracy and speed, nor can they cope with large-scale data. Security teams spend a lot of time updating rules to respond to pattern changes. However, the results are often unsatisfactory because dynamic data usage disrupts static rules.
Aspect | Traditional Data Security Solutions | Bedrock Solution |
Time Spent | Longer initialization time, requires additional tools or steps to ensure policy enforcement, and problem resolution. Rules need to constantly change to address different databases, data types, and threats. | Operational within minutes, seamlessly integrates with existing systems. AIR automates learning from data and business context. |
Deployment Mode | Exposes enterprise data to employees, complex deployment. | Bedrock does not access data in plaintext; one-click deployment. |
Data Visibility | Limited data visibility. | Intuitively displays relationships between data and services; demonstrates data authorization structures, and shows actual data usage. |
Data Classification | Relies on rules, complex regular expressions to achieve limited data classification. Data networks cannot effectively display. | Can classify based on regulatory categories, data topics, file types, etc. Allows classification based on the actual meaning of data, while also supporting custom data types. Can analyze data duplication through data networks. |
GenAI Capabilities | Limited | Can pinpoint data used for model training. Trust Boundaries ensures sensitive data is not used for training. |
Risk Assessment | Limited | Assesses the attack risk for each database, service. Provides visually intuitive “impact radius,” showing other affected systems once an attack is received. |
Table 1: Comparison between Traditional Data Security Solutions and Bedrock Solution
“Frictionless” Data Security
Bedrock Security has always emphasized providing “frictionless” data security products and solutions. How should we understand “frictionless”? We may define it from the following dimensions:
From the Organization Perspective
Chief Information Security Officers (CISO) no longer lag behind business objectives when seeking data use within business departments and boards. They also do not have to bear additional information leakage risks.
From the Security Team’s Perspective
By simplifying data access and protection, internal security teams, including security centers, governance, and risk compliance teams, can collaborate seamlessly.
From an Individual Perspective
Members of the security team do not have to spend a lot of energy on work order processing; compliance teams do not need to hold a large number of meetings, which do not hinder the execution of normal business operations; and members of the engineering team have a lower workload because data security has been simplified.
How Does Bedrock Achieve This?
Bedrock achieves this through its Artificial Intelligence Reasoning (AIR) engine, which enables sustainable discovery, management, and protection of sensitive data. AIR automatically understands which data is most critical to the enterprise, allowing companies to protect their most valuable assets without slowing down data growth or hindering the use of data to accelerate business success.
1. Accurate Data Identification
The first step in achieving “frictionless” data security is accurate data classification and the ability to provide visualization of data relationships. Every organization conducts risk assessments to understand vulnerabilities and remediation measures. This is part of meeting compliance requirements and addressing data leaks. Risk assessment must identify, assess, and prioritize risks, including those related to data storage, processing, and transmission within the enterprise. For many organizations, identifying all created, modified, and transmitted data in a complex distributed data environment is a huge challenge. Traditional solutions struggle to maintain visibility in these cloud platforms and third-party services, leading to gaps in data discovery and classification, increasing risks.
Many traditional solutions rely on static rules (also known as regular expressions) to identify and classify data, inevitably missing any content that does not conform to these rigid rules, leading to a large amount of data that cannot be identified and protected. Solutions that use “brute force” to traverse and review each line of a file for data classification can accurately assess data but are costly and take months to complete, resulting in outdated data. Simply sampling a small portion of data is fast but does not classify all data, making some sensitive information vulnerable. Furthermore, traditional solutions struggle to identify and classify unstructured data and cannot handle the exponential growth of data, affecting performance.
The Bedrock platform uses existing APIs to discover structured and unstructured data sets, providing comprehensive data insights, including data classification, context and data mapping, data types, and context. Bedrock dynamically adjusts sampling based on the characteristics of each specific file and data store. For example, for structured data sets like databases, Bedrock can analyze small samples and with a high probability determine the data types stored in the database, thereby accurately and quickly classifying data. For unstructured data, Bedrock uses larger samples to understand it. If the AIR engine identifies sensitive data in a specific folder, it increases the sample size to ensure that all sensitive data is identified and classified. The ability to adjust scanning based on data types and discovered content speeds up the data discovery process, ensuring the accuracy and visibility of all data.
In addition to finding data, classifying data, and determining how data flows, Bedrock uses Large Language Models (LLMs) and other AI and Machine Learning methods to analyze data and determine the data type, content, and owner of files. For example, a bank uses a customer’s social security number in account information, but also stores an employee’s social security number. Although these data types are the same, their purposes and ownership are completely different. The AIR engine helps to properly classify data and coordinate the permissions that need to be set based on the business purpose of the data.
2. Data Security and Compliance
Accurate classification of data types and purposes enables the Bedrock platform to generate risks and impact scores for each data volume and its sensitive data. This rating includes the impact on the business after data leaks, helping to determine the priority of risks. The visibility provided by traditional solutions is not comprehensive, making it difficult to uniformly enforce security policies or ensure the compliance of all assets. The AIR engine can identify and classify all data, then create impact scores based on the criticality of the data, informing which databases and data stores need the most protection. Effective data protection also requires continuous security assessments for real-time data detection and response to anomalies caused by changing data and threats. The Bedrock platform enables sustainable data discovery, analysis, and classification, allowing organizations to ensure prompt handling of any security or compliance breaches.
Compliance with regulatory requirements is important, but ensuring compliance with internal policies is equally important. The Bedrock platform allows customers to apply their own policies and restrictions, which are then analyzed to ensure compliance with their own data security requirements. Bedrock simplifies this process by providing “Trust Boundaries.” These boundaries provide a fast, adaptive, and automated way to define, remind, and control data violations based on critical business needs.
Bedrock’s Trust Boundaries allow policies to be written in natural language, providing an easy and flexible way to manage secure data access. The dynamic understanding of data by the AIR engine means that users do not have to worry about defining each data group but only focus on how to use this data and who can access it.
3. Reducing Data Exposure Risks
Based on its fast and accurate risk analysis capabilities, the Bedrock platform can understand data, manage policies and violations, thereby minimizing risks. It allows organizations to gain insight into existing issues in the environment and offers suggestions on how to address them. Bedrock reduces risks in the following three ways:
a) Reduce Data: Identify all owned data, determine which data is unnecessary, and delete or move it to cold storage, making it difficult to access.
b) Minimize Access: Access control is a key method to minimize data risks. Once data, how it flows, and who can access it are understood, it is possible to determine who needs the data and restrict access to those who really need it.
c) Strengthen Data: Encryption is a way to make data harder to steal, but to do so, it is necessary to continuously discover what data exists in the environment.
Bedrock’s AIR engine minimizes data exposure risks by reducing them to a minimum by identifying outdated or ghost data; it also assesses the impact of identity access to set permissions according to the principle of least privilege and strengthens data to minimize the impact of data leaks. The more users can access sensitive data sets, the more ways attackers have to invade accounts, eventually endangering the data itself. The Bedrock platform minimizes this risk by reducing data, minimizing access, and strengthening data, and easily remedying any problems that arise.
Bedrock’s Architecture
Bedrock comprises two key components: Bedrock Outpost Analyzer, deployed in the customer’s environment through infrastructure as code (IaC), and Bedrock SaaS Platform, which collects and processes metadata using Bedrock’s AIR Engine and provides the Bedrock user interface.
Bedrock Outpost Analyzer
Discovers and classifies data on the customer’s data side, sending only metadata to the Bedrock SaaS Platform. This means that Bedrock does not access the customer’s data, thus ensuring customer privacy. For example, if a database field is named “Passport Number,” Bedrock only captures the field name, not the actual value.
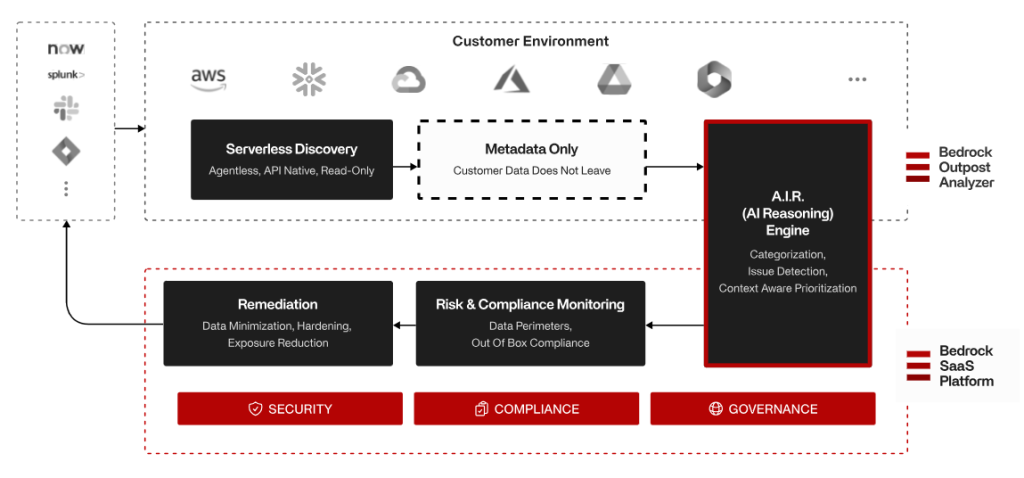
Serverless Discovery
Bedrock Outpost Analyzer uses existing APIs to discover data assets, supporting both structured and unstructured data. With simple Bedrock configuration, the system automates the rest of the process. Throughout this process, data remains within the domain.
AIR Engine
AIR is Bedrock’s core, capable of understanding the true meaning and business value of data. AIR processes metadata information from the customer’s environment, categorizes it, analyzes security risks from multiple dimensions, and determines priorities for handling based on risk factors.
Remediation
For security events and risks identified by AIR, Bedrock provides corresponding suggestions and remediation measures. Users can apply these measures with a single click using Bedrock’s API integration tools or generate work orders containing detailed instructions on how to address these security issues.
Risk & Compliance Monitoring
Bedrock can detect hundreds of behaviors that violate security standards, including improper configurations and excessive permission settings. Bedrock’s Trust Boundary helps customers quickly identify important data, ensuring its security. For generative AI, Trust Boundary can protect sensitive information, such as source and result sensitive information and core intellectual property information.
“Frictionless” Security Team Collaboration
Bedrock helps different teams collaborate efficiently on security. The security team can access security risk events; the compliance team can obtain compliance analysis reports, create new policies to address assessments and audits; and the governance team can create data access policies based on users, data types, data sources, and other factors.
Conclusion
In today’s era of cloud computing and AI, managing and ensuring data security is crucial for organizations. Traditional data security solutions cannot handle large-scale, dynamic data and are challenging to apply in cloud computing and AI environments. Organizations must take data security measures to efficiently and seamlessly implement risk management and remediation. Bedrock Security, as described in this article, achieves rapid and accurate risk assessment through AIR, ensuring efficient and secure data management for organizations even as data continues to grow. Bedrock’s AIR provides visual data correlation analysis, helping organizations reduce their attack surface.
Analyzing Bedrock Security and other companies in this year’s Innovation Sandbox, we can see that using AI to solve data security problems has become a trend in recent years. In NSFOCUS’s upcoming blog posts, we will also introduce technologies such as using AI to achieve hierarchical classification of sensitive data. Interested readers are welcome to continue following.
References:
[2] The Impact of Data Growth on Enterprises
[3] Cybersecurity Risk Management, Strategy, Governance, and Incident Disclosure