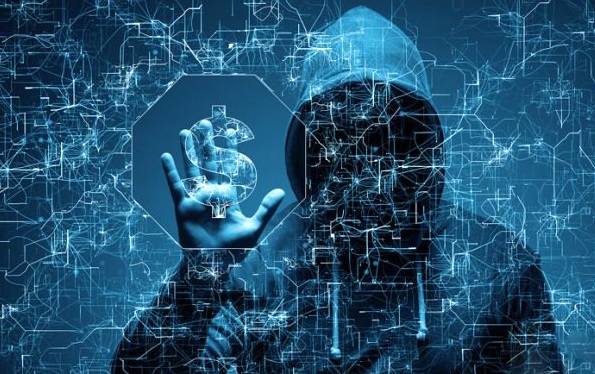
At RSA 2019, as indicated by Bugra Karabey, the senior risk manager from Microsoft, artificial intelligence (AI) and machine learning (ML) technologies have found a ubiquitous application in the cybersecurity field. Currently, ML is the most popular AI technology which is extensively used. Meanwhile, people begin to think about drawbacks and even security risks of ML.
Speaking at the RSA conference, Anthony J. Ferrante, an executive director of FTI Cybersecurity pointed out that currently, AI has taken such a great amount of our personal information that it can easily obtain our privacy, conduct product placement, gain our confidential access, and even seek to influence public opinion such as changing the way people cast a ballot.
Tienne Greeff and Wicus Ross from Secure Data pointed out that AI & ML is more suited to offensive than defensive applications. For example, attackers could use deep learning to bypass AI-based phishing detection, fool the image recognition system, and use reinforcement learning to launch web application attacks.
At RSA Conference 2019, Tao Zhou, a senior staff algorithm engineer from Alibaba Security, Alibaba Group, started his presentation on application of statistical learning to intrusion detection in the context of massive big data with an account of challenges facing Internet giants in security data analysis, and then shared his constructive ideas on this topic.
For starters, Tao Zhou pointed out that in security defense and attack, the largest advantage defenders have lies in massive data.
However, data is a double-edged sword. How to put massive data into good use is a common question baffling defenders in the era of big data.
Massive data is far beyond the human capacity to process. Therefore, it is high time for computers to step in, relieving people of the burden of complex computation with various algorithm models, as represented by machine learning and statistical modeling.
Machine learning, when applied as the only approach to intrusion detection, would encounter a lot of problems because machine learning is good at detecting “normal patterns”, but intrusion is abnormal behavior. Moreover, for supervised learning, machine learning algorithms need large amounts of labeled data, which are hard to obtain. For unsupervised learning, currently the accuracy and recall rate of almost all related algorithms cannot support security operations. Finally, the biggest problem of machine learning is that the classification model built on data training is usually too complex to interpret. The only answer of “Yes” or “No” is insufficient for security analysis.
Therefore, when applying machine learning to intrusion detection, we usually need to specify certain scenarios, typically those where labeled data is easy to obtain, like spam detection, DGA domain detection, and web crawler detection.
During attack modeling, a model should be built on preprocessed data to reflect a specific type of attack. The same type of attacks tends to have common features, which can be obtained from data of abnormal behavior. In this step, precision is the most important indicator, reflecting an emphasis on the accuracy of determination of attack patterns.
The kill chain-based model is a good option for modeling in this step.
Sum-up
In intrusion data analysis, it is a must to interpret analysis results. Traditional machine learning algorithms built upon training data, however, are usually “black boxes” that deny interpretation.
For this reason, many scholars begin to study the explainable AI (XAI), with a purpose of delivering interpretable analysis results, whether using the statistical modeling (for example, topic modeling frequently mentioned at this year’s RSA conference) or the knowledge atlas (semantic network) technology (such as Watson from IBM).