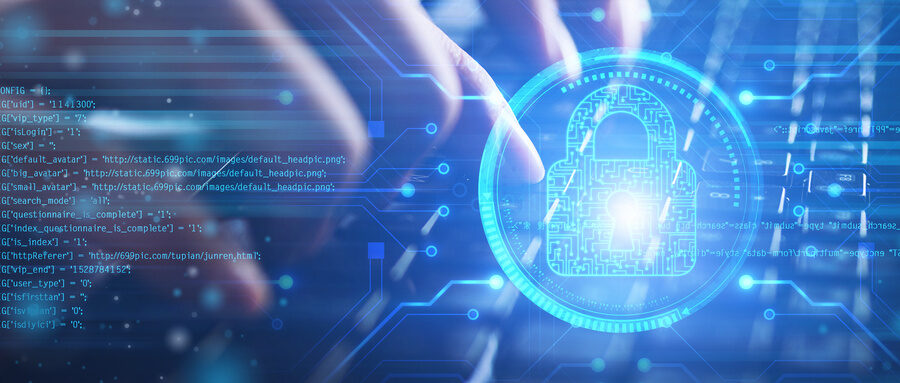
Compliance has seen radical changes in the requirements and driving force of data security and a broader category of data objects under data security protection. Application scenarios covered by data security will become more diversified, and data security requirements will cover all phases of the data lifecycle. In order to better cope with the challenges posed by compliance, enterprises need to transform from traditional single-point development to systematic data security development.
This post first analyzes the compliance requirements and security challenges of three typical scenarios, i.e. intelligent identification and classification of sensitive data, assessment of residual risks in masked data, and detection of abnormal data operations. Then, the subsequent sections describe three types of technologies to address these challenges, including intelligent identification of sensitive data, risk assessment of masked data, and user and entity behavior analytics.
Intelligent Identification and Classification of Sensitive Data
In data security governance within enterprises, the first step is to accurately and comprehensively identify sensitive data in systems and applications.
- GDPR Compliance Requirement
GDPR protects personal data. Personal data is broadly defined and includes basic personal information such as the name, age, gender, personal photos, IP address, MAC address, and Internet cookies (Article 4).
- Problem and Challenge
Traditional methods for identifying sensitive data require manual design of rules and dictionaries, which may not be inclusive enough to cover all sensitive data.
Solution: Intelligent identification of sensitive data
Assessment of Residual Risks in Masked Data
Data masking has been widely used by enterprises. Security effects of different masking methods need to be quantitatively assessed.
- GDPR Compliance Requirement
During data processing, appropriate technical measures should be used to reasonably deal with security risks (Article 32).
- Problem and Challenge
How to characterize residual privacy risks of masked data from the perspective of attacks.
Solution: Assessment of residual risks in masked data
Detection of Abnormal Data Operations
In security protection of databases and big data platforms, normal and abnormal behavior patterns need to be recorded and analyzed.
- GDPR Compliance Requirement
See the compliance clause in section 3.1.2.
- Problem and Challenge
Common anomaly detection methods based on rules and thresholds cannot cope with the challenges posed by complex services.
Solution: User and entity behavior analytics
Below illustrates each technology for solutions above in details.
Intelligent Identification of Sensitive Data
Intelligent identification of sensitive data is mainly used in unstructured data types, such as texts and images. It includes three types of intelligent algorithms: similarity-based algorithms, unsupervised learning algorithms, and supervised learning algorithms.
Similarity-based algorithms can accurately detect unstructured data stored in the form of documents, such as Word and PowerPoint files and PDF documents. The main idea is to extract the fingerprints of documents that contain sensitive information and those of documents that are to be detected, and then make comparisons via similarity algorithms to confirm whether the detected documents contain sensitive information according to the preset similarity thresholds.
Unsupervised learning algorithms require no manual labeling. After extracting the features of sensitive data to be detected, use clustering algorithms such as K-means and DBSCAN to cluster the input sample vectors to form a dataset of different “clusters”. Then, some samples of these “clusters” are analyzed to determine whether they are sensitive or non-sensitive.
Supervised learning algorithms require collection of a certain amount of training data (such as documents and pictures), and the data is manually labeled as sensitive or non-sensitive. Appropriate supervised learning algorithms are selected, such as the support vector machine (SVM), decision tree, random forest, and neural network. Then, model training and parameter tuning are performed on the training data. After the training is completed, apply the output model to the new data for intelligent identification and prediction to automatically output data types as sensitive or non-sensitive data.
Risk Assessment of Masked Data
Risk assessment of masked data analyzes and depicts privacy breach risks of masked data. The technology can be classified into two categories: qualitative judgment methods based on manual spot checks and general assessment techniques. Qualitative judgment methods based on manual spot checks refer to expert inspection and judgment in accordance with standard procedures and forms. However, such methods are very expensive. General assessment techniques and data masking methods have nothing to do with models, and are usually called re-identification risk measurement in academics. Canadian scholar El Emam developed a more general re-identification risk assessment theory and method and divided attacks into three types of scenarios according to the attacker’s ability and attack intention. Besides, he vividly named attacks Prosecutor attack, Journalist attack, and Marketer attack [i]. In these three attack scenarios, El Emam et al. designed a set of evaluation metrics system based on probability and distribution. The system includes eight types of metrics, which can respectively describe risk information such as the average re-identification probability, the highest re-identification probability, and the proportion of records with a high probability of re-identification. Their numerical range is [0,1]. 1 means the highest re-identification risk, and 0 means almost the lowest re-identification risk. In specific applications, appropriate metrics should be selected for re-identification risk assessment based on actual conditions.
User and Entity Behavior Analytics
User and Entity Behavior Analytics (UEBA) can promptly detect and identify attacks and abnormal behaviors from massively collected security data through continuous profiling and modeling of user entities. UEBA includes both basic analysis methods (such as threshold analysis) and advanced ones (such as correlation-based analytics and machine learning).
- Threshold analysis: mainly detects anomalies on the basis of statistical methods. It performs statistics on the data over a period of time and compares the data with thresholds. If the data exceeds the threshold range, it is deemed abnormal. For example, the statistical values of normal historical inbound and outbound traffic are used as thresholds to identify abnormal behaviors.
- Correlation-based analytics: used to discover the meaningful relationships hidden in large datasets. You can conduct correlation-based analytics based on algorithms to dig out association rules between data. In addition, you can use tools such as graph databases to dig out associations between data.
- Machine learning: Constant learning of continuous evolution of a large quantity of historical data enables you to detect and identify abnormal or malicious behaviors and is advantageous especially in detecting unknown threats of data security. UEBA can apply logistic regression, SVM, K-Means clustering, DBSCAN density clustering, random forest, and other algorithms.
UEBA has been applied in data security, typically in the detection of anomalies in database breaches. Focusing on sensitive data, it collects information about data operations by user entities, establishes behavioral baselines of multidimensional entities through the process of data analysis and learning, uses machine learning algorithms and predefined rules to find abnormal behaviors that seriously deviate from the baselines, and promptly discover such violations as data theft by insiders and partners. In this scenario, the 5W1H (who, when, where, what, why, how) model is usually used for UEBA analysis and modeling. Through the analysis of entity behaviors from six dimensions, data breaches and abnormal operations can be discovered in time.
Data security governance within enterprises is to meet both the requirements of compliance and the needs of internal security. Security of sensitive data is the top priority in enterprise data security protection. Identification of sensitive data is an important scenario. For unstructured or semi-structured data, such as documents, web pages, and XML texts, intelligent technologies in sensitive data need to be applied to facilitate machine learning and natural language processing to improve the identification accuracy. As a mature technology, data masking has been widely used in the processing of sensitive data in enterprises. However, data masking does not completely eliminate privacy risks. After masking is completed, the residual risks of the masked datasets still need to be assessed and detected for risk perception and control. Flows and operations of sensitive data need to be monitored and audited. As a data-driven intelligent technology, UEBA can intelligently discover abnormal user data operations and attack events. These innovative technologies improve data security governance and protection within enterprises.
Related posts:
Compliance-driven Data Security
Cutting-Edge Technologies Empowering Security and Compliance of User Privacy Data