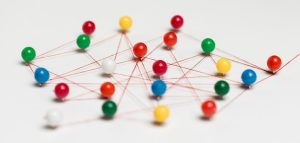
The security knowledge graph, a knowledge graph specific to the security domain, is the key to realizing cognitive intelligence in cyber security, and it also lays an indispensable technological foundation for dealing with advanced, continuous and complex threats and risks in cyberspace. NSFOCUS published a series of articles about the application of the security knowledge graph in several scenarios. This article focuses on the application of the security knowledge graph in the integration of functional safety with information security in the industrial control system.
Background of the Integration of Function Safety and Information Security
As a new generation of information and communication technologies gradually becomes mature, emerging technologies like intelligent manufacturing, industry 4.0, industrial cloud and IoT develop continuously, and industrialization and informatization are highly integrated, the modern industrial control system is gradually transformed from the traditional stand-alone, closed mode to the interconnected, open and intelligent mode.
Such transformation in the industry not only improves production efficiency but also opens up more information interfaces. The industrial control process can be connected to the internal IT network and the external Internet, with IT technologies introduced into all aspects of production and manufacturing, and the boundaries between IT and OT environments are becoming blurred. In such a complex and changing industrial control network environment, represented by Stuxnet, attackers can use the coupling relationship between information and physical systems to formulate corresponding attack strategies for specific business processes and security plans, and disrupt the normal operation of the system. The traditional industrial control security protection system designed for the sole purpose of information security or functional safety cannot comprehensively describe, detect and defend against such new attacks.
The modern industrial control system has the characteristics of a typical cyber-physical system (CPS), which is a multi-dimensional complex system comprising computing, networking, control, and physical environments. Its own complexity and the diversity of the industrial site environment determine that there are various sources of industrial security risks, and the comprehensive identification, analysis and management of system security risks are required. The future industrial security protection tends to integrate functional safety with information security, with the aim and within the constraint of the availability of business system functional safety, and with the attack threat in network information security into consideration. The optimized design of the system with two types of security protection capabilities settles conflicts, realizes the integration of functional safety and information security for the industrial system, and reduces the information security risks brought by the CPS.
Transformation of the industrial system security protection system
In view of the new characteristics of the CPS, different countries have begun to set out requirements in their standards and guidelines for integrating functional safety and information security in industrial systems. According to the GB/T 30976 Industrial Control System Security, information security, physical security and functional safety may be closely related. In some cases, other security measures may provide an independent protective layer for information security, and their integrity may also be undermined by additional information security measures. Therefore, the potential interaction among the three and their consequences should be considered in specific risk assessment activities. The NIST-SP800-82 Guide to Industrial Control Systems (ICS) Security published by the National Institute of Standards and Technology (NIST) sets out clear requirements for risk management: ” The personnel responsible for operating, securing, and maintaining OT must understand the important link between safety and security.” The ISO 26262:2018 Road Vehicles Functional Safety Standards added requirements for interactions between functional safety and information security in functional safety management, concept, product development, and production and operation phases.
Difficulties in the Integration of Industrial Security and Safety
In the actual industrial field environment, the functional safety protection system represented by the safety instrumented system (SIS) senses specific physical parameters (temperature, pressure, distance, location, etc.) using sensors, and the logical unit calculates and compares the detected parameter values, thus determining whether the status is normal. The information security protection system represented by the intrusion detection system (IDS) conducts online monitoring of network transmission packets or host status information, extracts and analyzes relevant data features, and identifies abnormal data. Although both provide massive monitoring data for system analysis, a single detection result is not enough to provide a complete picture of the security situation of an industrial system. Technical solutions with relevant context are needed to quickly understand the overall situation behind a series of alarms, so as to avoid wasting time on isolated nodes and save more time for risk mitigation decisions and actions.
At present, the following problems still exist in the integrated protection of industrial safety and security:
One is the information island. In the industrial environment, information security and functional safety have established security protection systems based on IEC 62443 and IEC 61508 respectively for different sources of system risks. The two systems have not formed an effective knowledge link to break down the “information island”, so it is difficult to comprehensively analyze the impact of industrial systems in the attack process.
The second is data waste. In the information layer and physical layer of the industrial control system, various security systems generate massive amounts of monitoring data every day. These data sources are scattered, semantically diverse, heterogeneous and interrelated, and the operational behaviors of attackers are hidden in them. There is still a lack of mining and presentation of the data associated with attack events, which does not help adopt correct and reasonable security strategies.
The third is low efficiency. Against the background of increasingly complex industrial production equipment, the traditional method of manually analyzing security issues with the help of security experts is time-consuming and difficult. Therefore, deep learning, AI modeling and processing, and knowledge processing need to be introduced into this field, so as to simulate relevant experts by computer for security analysis on the system, and achieve rapid reasoning and location of system security risks.
Applications of the Security Knowledge Graph
With the further integration and development of informatization and industrialization, industrial security protection is faced with the situation of diverse, complex and isolated data, as well as the low value of single data items. Meanwhile, there are security analysis requirements such as relationship search, alarm processing, and risk prediction. With the development of industrial production, the methods and technologies of system security analysis are also constantly improving, which can be roughly divided into three stages. The first stage is to process raw knowledge relying on the experience of security analysts and instrument judgment. In this stage, domain experts or maintenance personnel monitor the status of field equipment, and manually identify and locate hazard sources based on personal experience and the characteristics of the equipment running status. In the second stage, with the improvement of information transmission and processing technology, and the combination of sensors and computer technology, technicians can conduct an automatic security analysis of equipment online, but human participation is still required for identifying and processing system risks. In the third stage, against the background of increasingly complex industrial production equipment, the previous security analysis technology can hardly solve various problems in the operation of the system. Therefore, methods based on deep learning and AI need to be introduced into this field, so as to simulate relevant experts by computer for security analysis on the system, and achieve rapid reasoning and location of system security risks.
As an important branch technology of AI, the knowledge graph is very suitable for analyzing data extracted from unstructured resources, including implicit metadata providing structure and content. It is often used to describe the relationships between various entities or concepts in the objective world. The security knowledge graph stimulates the process of structuring heterogeneous data, allows various security data to be connected, and provides core support for the intervention of various security algorithms, thereby enabling knowledge extraction, fusion, analysis, inference, and decision-making capabilities in the security field.
Application of the security knowledge graph in the integrated protection of industrial system safety and security
In the scenario of integrated protection of industrial system safety and security, the security knowledge graph employs big data analysis and graph mining technologies to analyze the coupling relationship between the information layer and the physical layer in the modern industrial control system in depth, which can comprehensively show the variables and their relationships in the operation process of the industrial control system. As the infrastructure for intelligent knowledge applications, the knowledge graph can automatically collect industrial process information from multiple heterogeneous security data sources, and integrate known functional safety and information security knowledge into a data model, which allows the security system to employ the reasoning technology of the knowledge graph for comprehensive analysis. The security analysis results of the knowledge base allow predicting the hazards in the business process, and provide a query mechanism to help security management personnel grasp security dynamics, make timely decisions and responses, provide intelligent assistance in and automate capabilities of “decision making, risk prediction, accident analysis, and attack identification”, and provide intelligent security protection with “comprehensive protection, intelligent analysis, and automatic response.”
Conclusion
The knowledge graph is specialized in analyzing problems from the “relationship” perspective, and making judgments on the security situation of the system by associating the alarm data of the industrial operation process. Compared with the traditional industrial security risk analysis that relies on the participation of personnel, the application of the knowledge graph has made significant progress in the scope of data analysis, depth of data mining, and visual data analysis, which can describe the risk relationship between the physical layer and information layer of the industrial system more accurately and quickly, make timely risk predictions based on the analysis results, and formulate relevant countermeasures to ensure the secure and stable operation of the industrial process.
Posts about Security Knowledge Graph:
- Security Knowledge Graph | Technologies and Applications of the Security Knowledge Graph
- Security Knowledge Graph | Build an APT Group Graph to Avoid the Information Island Effect
- Security Knowledge Graph | APT Group Profiling and Attribution
- Security Knowledge Graph | Cyberspace Mapping Strengthens Tailor-made Security