In a recent achievement, the paper BABD: A Bitcoin Address Behavior Dataset for Pattern Analysis, a collaboration between the NSFOCUS research team and Professor Ren Wei’s team at the Computer School of China University of Geosciences, has been featured in the prestigious journal IEEE Transactions on Information Forensics and Security (TIFS).
IEEE Transactions on Information Forensics and Security (TIFS) is a top-tier journal in the field of information security, classified as a Tier 1 journal by the Chinese Academy of Sciences JCR, recommended by the China Computer Society as a Class A international journal in network and information security, with a current impact factor of 6.8.
The first author of the paper is Xiang Yuexin, a Master of Engineering at the Computer School of China University of Geosciences. The collaboration members include Liu Wenmao, Director of the Innovation Center and Head of Xingyun Lab at NSFOCUS, with Professor Ren Wei from the School of Computer Science of China University of Geosciences as the corresponding author.
With the widespread adoption of cryptocurrencies, such as Bitcoin, in the financial and online service sectors, their characteristics of anonymity and decentralization have facilitated technological innovation and transaction convenience. However, they have also brought potential risks, especially in illegal transactions. The paper addresses this dual-edged sword by constructing a dataset called BABD-13, containing 13 types of Bitcoin addresses, 5 categories of indicators, and 148 features. This dataset is currently the largest publicly available dataset of Bitcoin address behavior. The paper also introduces a novel subgraph generation algorithm, BTC-SubGen, for extracting subgraphs corresponding to Bitcoin addresses from the Bitcoin transaction graph and analyzing the structural features of local transaction subgraphs. The paper applies five common machine learning models to perform classification tasks on BABD-13, achieving accuracy rates ranging from 93.24% to 97.13%. Additionally, the paper delves into the behavior patterns of different types of Bitcoin addresses, revealing meaningful patterns. This research aims to enhance the monitoring capabilities of illegal cryptocurrency usage, thereby strengthening the security and stability of the entire cryptocurrency ecosystem.
This collaboration between NSFOCUS and Professor Ren Wei’s team at China University of Geosciences is supported by the CCF-NSFOCUS Kunpeng Fund (CCF-NSFOCUS2021008). The results are expected to contribute to the construction of labeled datasets for typical digital currency transactions, pattern analysis, and secure supervision of digital financial transaction behavior, especially in the areas of fintech security and cybercrime investigation, such as money laundering, underground gambling, and illegal fundraising.
Paper Information:
Title: BABD: A Bitcoin Address Behavior Dataset for Pattern Analysis
DOI: 10.1109/TIFS.2023.3347894 Paper Link: Read the paper
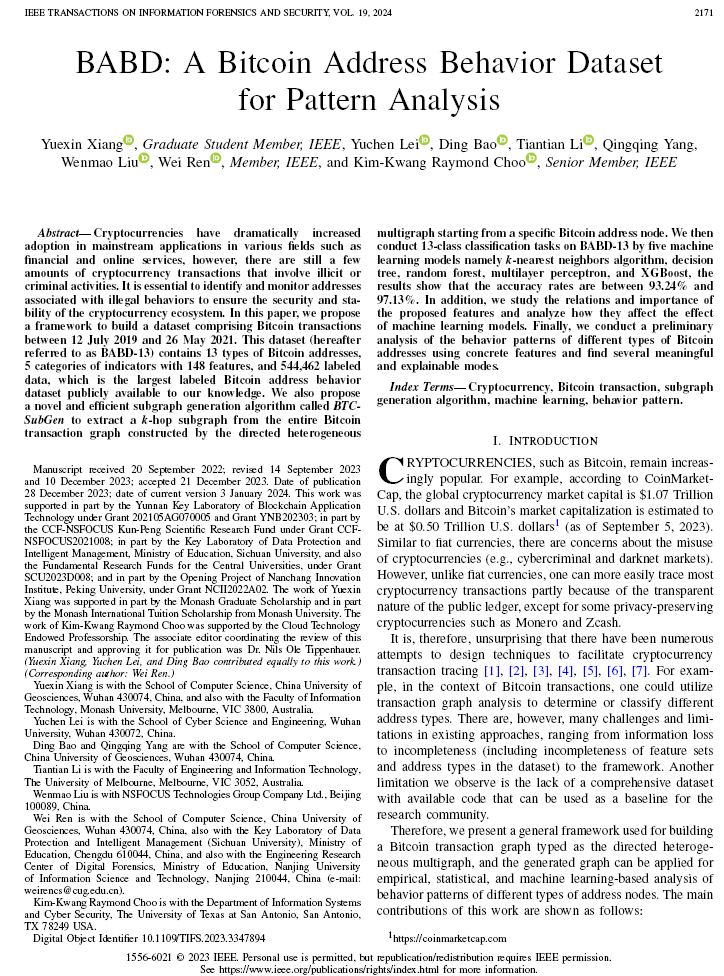